Mastering AI Projects - Preventing First-Time Mistakes
The road to successful AI integration is packed with challenges, particularly for those companies trying it for the first time.
This blog post is part of a three-post series dedicated to helping companies avoid three significant mistakes when starting AI initiatives and build on my experience.
I first started building machine learning-powered products back in 2013. In the past ten years, I have worked on delivering more than 50 AI-powered projects for companies in the HR Tech and Online Recruiting industry like Experteer GmbH, Jobware AG, Jobiak, Jobiqo, Getin GmbH, Ofir, Jobsync, my job boards - Crypto Careers, Web3jobs, GetinAI and many more.
In the first blog post, I will explore one of the most prominent mistakes when organizations dive into AI. This critical oversight can derail even the most promising projects - the need for a dedicated team. Using a real-life example from my career, I aim to highlight the consequences of this oversight, discuss its impact on project success, and provide practical advice to help you avoid similar pitfalls.
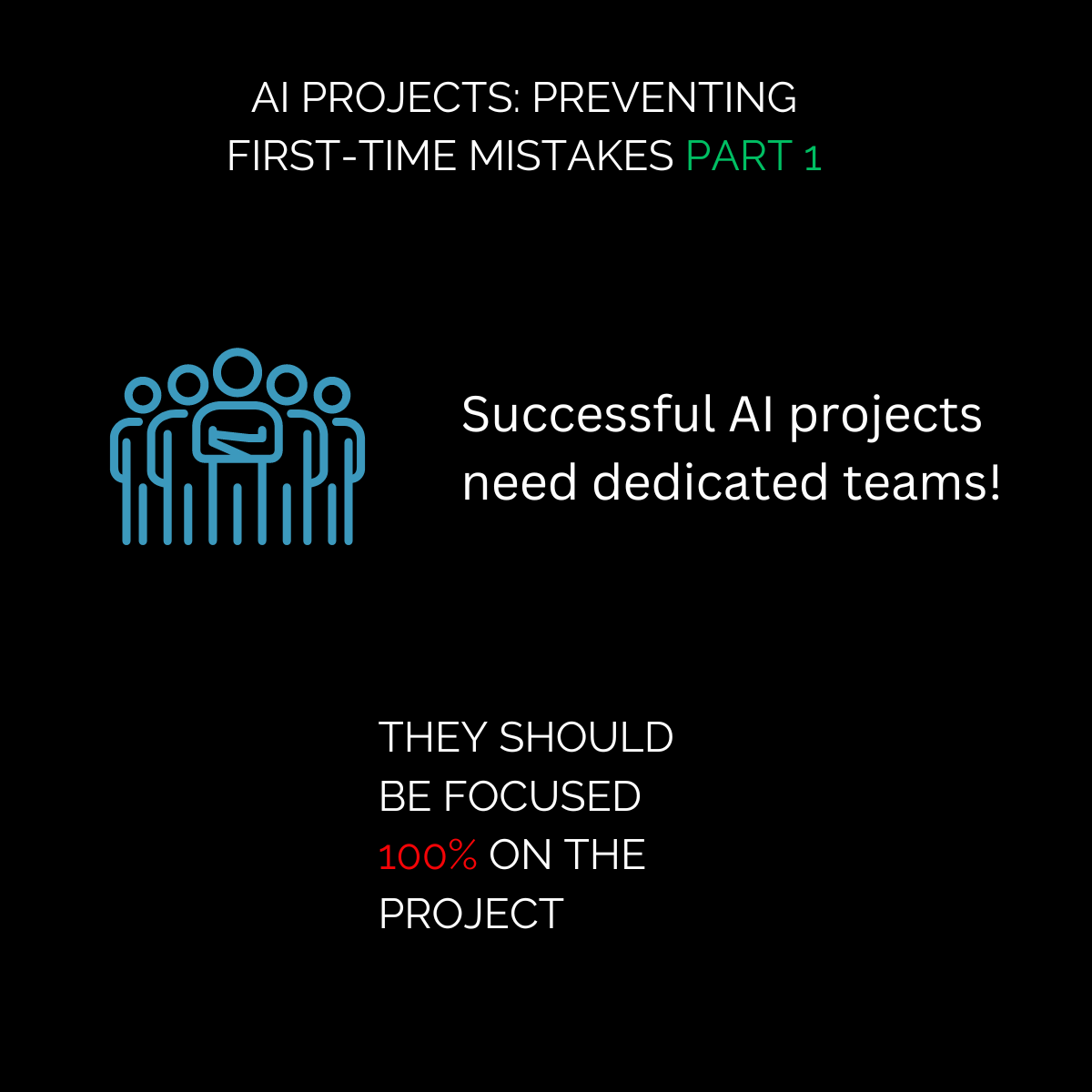
My experience with large AI-initiatives
Years ago, I had the chance to lead a significant AI initiative. The team had no experience with machine learning, ML Ops or anything data-driven. It was a green field.
Back then, I was already part of the organization and had operational and managerial responsibilities - It was a real full-time job.
Now, the AI initiative planned was massive. It was first an R&D project to show that the technology could handle the use case we worked on back then. And even if the POC worked, we would have had to rebuild the existing data processing infrastructure to accommodate the ML services.
When I agreed to lead the initiative, I thought my responsibilities would be delegated, and I could work exclusively with the two developers on the AI initiative. This would have been an ideal scenario and the most logical one.
However, the CEO decided we should do it on the side, as part of our daily jobs – keep all operational and managerial responsibilities and do the AI initiative in parallel.
I was motivated to do it back then because it meant exposure to an entirely new technology and an exciting task, so I said yes. How bad could that be?
Not having a dedicated team – the results
Well, in short, we managed to deliver what we intended to.
Looking back on my experience today, I realize it was a terrible decision. There is the stress of doing two jobs, but I am not here to discuss the workload, only the strategic risks.
First, the project took a lot longer; we could have finished in 1/3 of the time if we did not have to be defocused from our daily jobs.
Second, because the project took a lot longer, we slowly lost support and buy-in from the rest of the management and the whole company.
The mood in the company changed from being very supportive and excited to skeptical and hostile towards our initiative.
Everything we were doing was reviewed constantly and questioned. This almost killed the initiative, which then already cost $1.5 million. So, to save $350K (the cost to cover the three of us), we almost lost 5x the investment. Now, this number is a lot higher. Think about all the meetings we had with stakeholders during the development cycle. Hours of time with team leads, and managers would have gone to waste. The indirect cost was another $500K.
Even worse, this initiative became a massive success for the company. The investment was paid off from cost savings in less than two years. The automation enabled the creation of new revenue models that returned the original investment in just five years. All of this future value was put at risk.
Not to mention the personal stress the team went through; we always have to defend what we do and explain why it takes so long.
Last but not least, once we were ready, the department that was supposed to take over the automatization process and support the roll-out was anything but friendly. They were skeptical because everything took so long, and during the testing phase, they saw results that were way ahead of what was initially promised.
What happened was that they became cautious in reviewing the predictions the system generated, even in cases where the accuracy did not call for it. As a result, the final rollout could have been shorter.
AI Projects are Change Management Projects
The major takeaway is that AI Projects are change management projects. Your initiative will likely only succeed if the team buys into what you want to roll out.
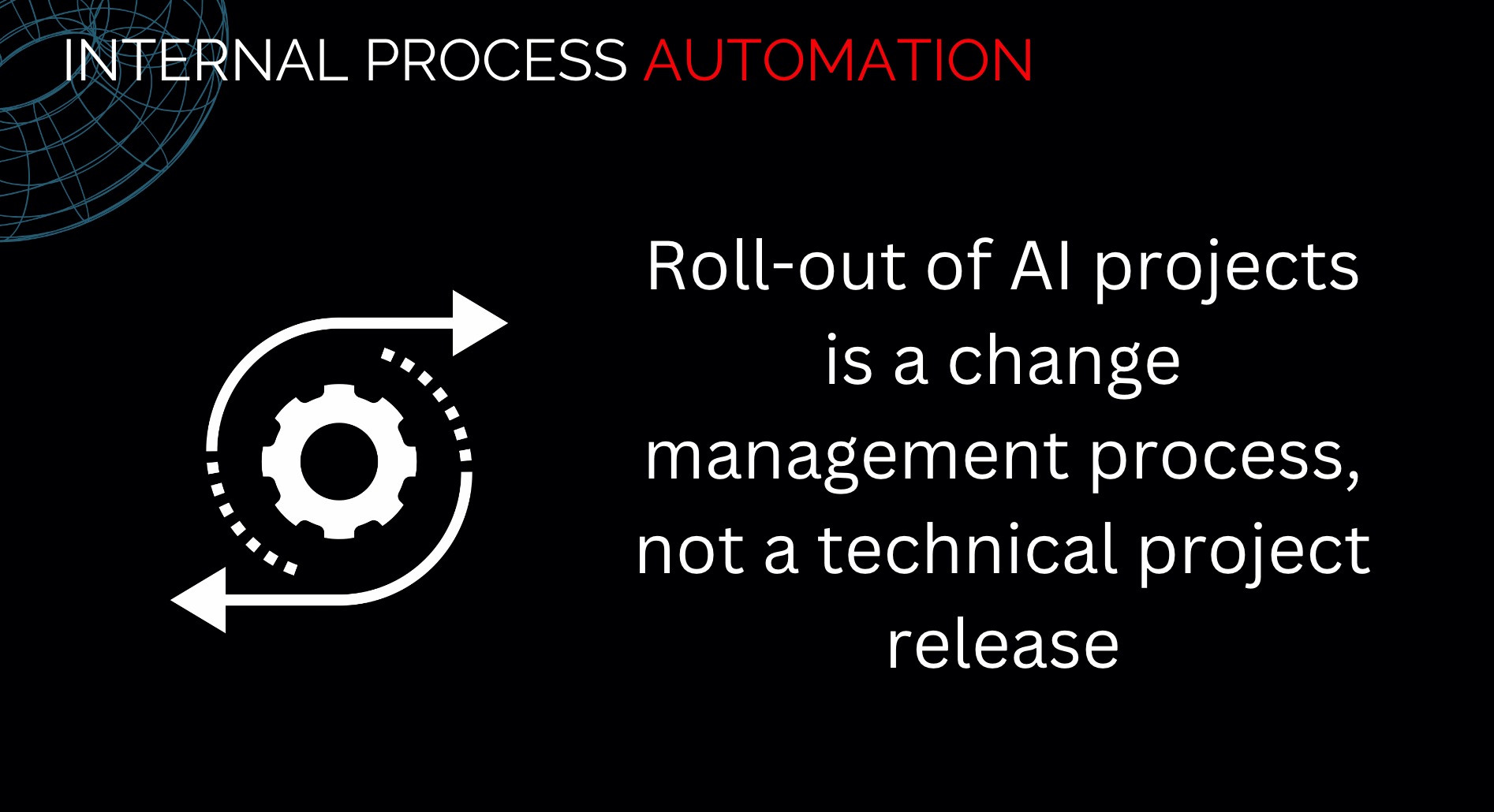
For your team to buy into what you want to build, you have to ensure the chances of success are very high.
So, the biggest mistake you can make when setting up AI initiatives or building AI-powered features for the first time is not setting up a dedicated team for this task, because your success chances will be lower and thus your change management process might suffer.
Conclusion
The primary takeaway from this experience is unmistakable: the necessity of a dedicated team when building AI-powered products, especially if this is your first one as a company. Without this, an AI project risks prolonged timelines, diminished support, and increased skepticism—all of which can jeopardize its success.
What does that mean for you if you find yourself in my spot?
1. Advocate for dedicated resources: make a compelling case to your organization's leadership about needing a dedicated team, emphasizing the potential return on investment and strategic advantage. Ensure you and your team are freed from other responsibilities to focus solely on the AI initiative.
2. If you are the one making the decision, do it. Give your team the freedom to work exclusively on AI projects, and don’t let them be split between multiple responsibilities.
3. As the AI initiative owner, establish clear goals and milestones: Set specific, measurable objectives and timelines for your project to maintain momentum and alignment across the team.
4. Communicate regularly with all stakeholders and keep them informed with regular updates. This transparency builds trust and maintains organizational support.
5. Prepare for change management: recognize that introducing AI into operations is not just a technical challenge but also a change management one. Plan accordingly.
By applying these steps, you can significantly enhance the chances of your AI project not just surviving but thriving.
Join the conversation
Are you planning or currently managing an AI project? I want to hear from you! Reach out on LinkedIn or share your experiences, challenges, and successes in the thread below to help build a community of practice:
Related reading
As someone involved in AI product development for ten years, I've observed four distinct phases of AI evolution. ChatGPT represents the latest phase in broadening AI's reach to businesses and consumers. It's important to note that these four waves are based on my personal experience and need to be academically recognized definitions.
In this article, you will learn what the four AI Waves mean and how ChatGPT will accelerate AI use in businesses.
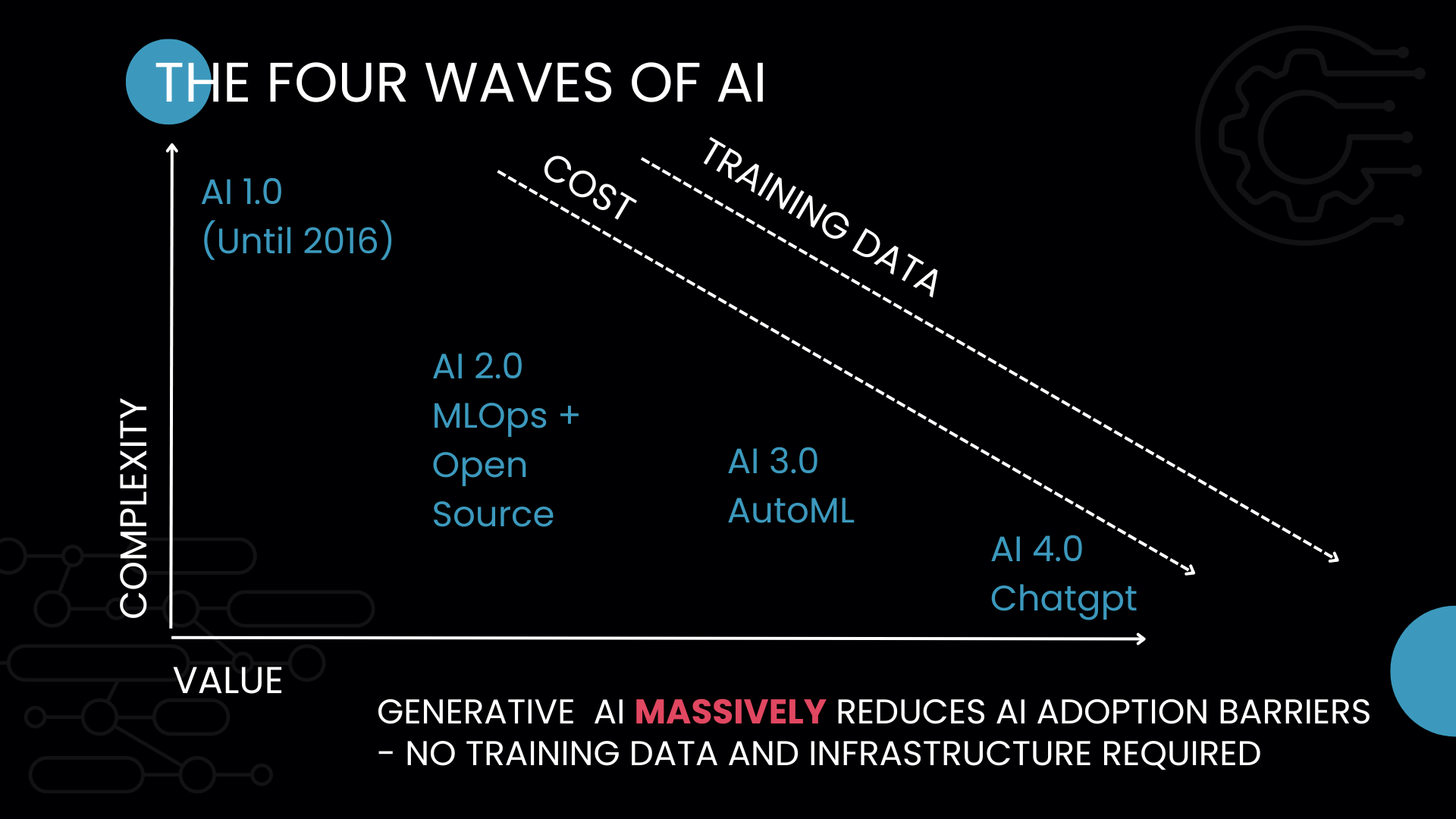
Did you like this post? Subscribe below and be the first to read the next post in the series!